Customer Sentiment AnalysisListening to Your Consumer Base
Customers are frequently leaving reviews to describe their consumer experiences, which can be key indicators of what specific problems a store is having. Being able to comprehend thousands of reviews through customer sentiment analysis can help identify patterns and behaviors to help improve your store’s performance.
Article originally published June 2022
Changing Business Environment
In the current digital age, the need to understand consumer behavior, analyze customer feedback, and use those insights to transform your current processes, are critical to delivering an exceptional customer experience. More and more businesses are using the digital interactions performed by consumers to improve customer satisfaction and increase engagement. The methodical approach used in this process relies on AI-enabled customer sentiment analysis. This involves customer feedback analysis which is based on understanding consumer behavior and using AI at every step of the process to quickly improve the quality of your service for your customers.
Converting Reviews into Insights and Actions
When you have a negative experience at a consumer location, what is one of the first thoughts that crosses your mind? For many, it’s to leave a negative review about that location detailing your experience. This doesn’t only serve to vent frustration but also works as an attempt to be heard in hopes that others will avoid the same experience. However, if a problem persists, more negative reviews will flow in, which can result in:
With hundreds to thousands of reviews being submitted weekly, it may seem daunting to try and understand what customers are saying without reading every single review. This is where sentiment analysis, a machine learning method, can be of great use.
At the most basic level, the process of customer sentiment analysis includes deconstructing a review into individual words, assigning applicable words a score to reflect how positive or negative that word is, and then aggregating the scores for each word to receive an average sentiment score for the review.
An Example of Sentiment Analysis
In this example of customer sentiment analysis, it is necessary to have a Lexicon. A Lexicon is simply a dictionary with key words and corresponding scores. So, if we set our score range where -5 means the word is very negative, and +5 where the word is very positive, we can create a simple Lexicon for this review that would look like this:
Word | Sentiment Score |
---|---|
Terrible | -4 |
Poor | -2 |
The other words in the review such as “the”, “and”, and “was” are filler or stop words. They do not need a score because those words have neither positive or negative connotations, and are just part of speech. Now that the sentiment scope is defined, the review needs to be broken up by word and joined with the Lexicon table. This would look something like:
Word | Sentiment Score |
---|---|
The | |
Food | |
Was | |
Terrible | -4 |
And | |
The | |
Service | |
Was | |
Poor | -2 |
From there, the sentiment score for the review is calculated as the average of the sentiment score values ((-4+-2)/2), which results in a final score of -3. On a scale of -5 to +5, -3 is a fairly negative score, which seems appropriate for the review which has been analyzed.
By utilizing machine learning, masses of reviews can be instantly assessed and scored, resulting in a quantifiable way to utilize and analyze review data that seemed unquantifiable before. Now with this data, we can begin to visualize and gain insights into how a store location may be performing, such as observing how the sentiment score changes week by week.
Word Clouds Reveal the Big Picture
It can also be useful to analyze the words from the reviews, in order to quickly identify any specific problems a location may be having. Word clouds are visualizations that display words and word frequency to gain an understanding of what specifically consumers like or dislike about a location.
After assigning scores to each word, an extra step in the analytic process can yield a word cloud that displays the most frequently used words. In this example, we used a public data set from a restaurant. The words shown below are the most common words used in positive and negative reviews, with the size of each word corresponding to how frequently it is used.
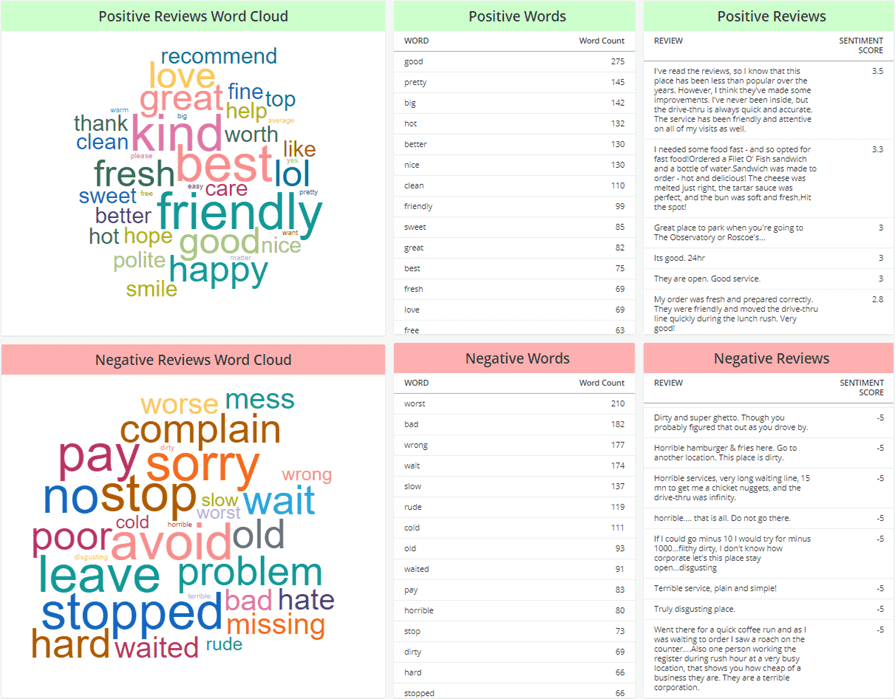
From the positive word cloud, it can be observed that this location does a great job at being kind and friendly, as those are the largest words in the cloud. However, knowledge gained from the negative word cloud may be more insightful. From here, it can be observed that some of the larger words include “wait” and “stopped”.
If people are using the word “wait” in their reviews, it may indicate that many customers at this location have to wait a significantly longer time to get their food. The same inference cannot be made for the word “stopped” however. So in this case, it would be helpful to use the word cloud as a filter to retrieve the reviews that have the word “stopped” in them to see what problem exists. It could be that employees are frequently sidetracked, distracted, or stopped helping customers.
Integrate Net Promoter Scores: Connect with Revenue
Customer sentiment analysis data can not only help understand these reviews, but also aid in gaining insights into revenue. Take for example the following visualization, which factors in revenue and net promoter scores:
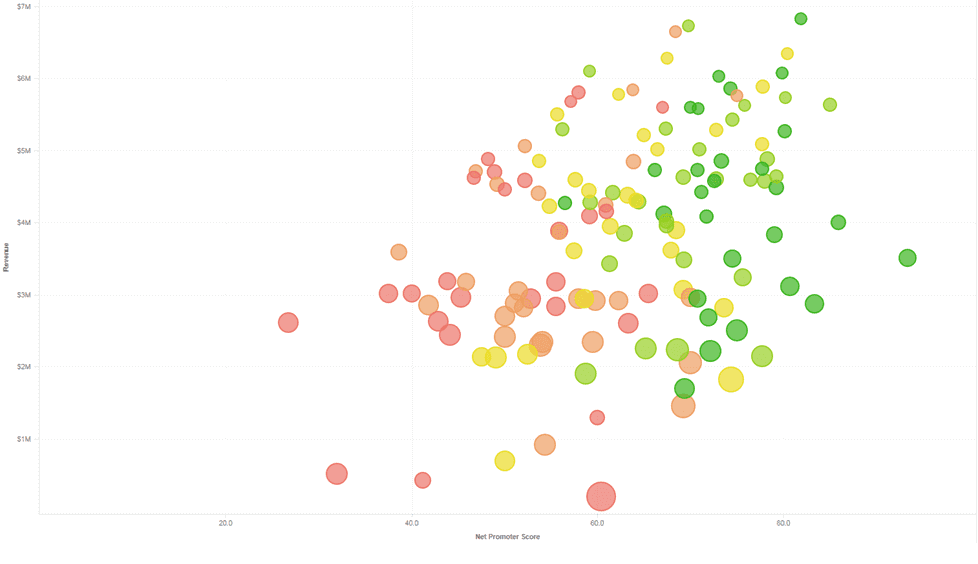
Many businesses use a survey with a series of questions that is used to create a net promoter score. This serves as an indication of how a customer’s experience with the service of a business was. Each plot point in this visualization represents a store, with the color representing the average sentiment score of that store.
From this, we can see that stores with low net promoter scores and generally lower sentiment scores tend to have less revenue than those with higher scores. A weekly analysis here could reveal any low performing stores, from which the reviews can be further analyzed to detect any specific problems and rectify them. Using reviews from the customers, stores can boost their revenue and profitability.
Outside of analyzing reviews as a whole, it may be beneficial to look at individual words too. Being able to search for specific words to see where and how often they are occurring can help detect problems. For example, to see how many locations may be having an issue with rudeness or slow service, using a network visualization yields the following:
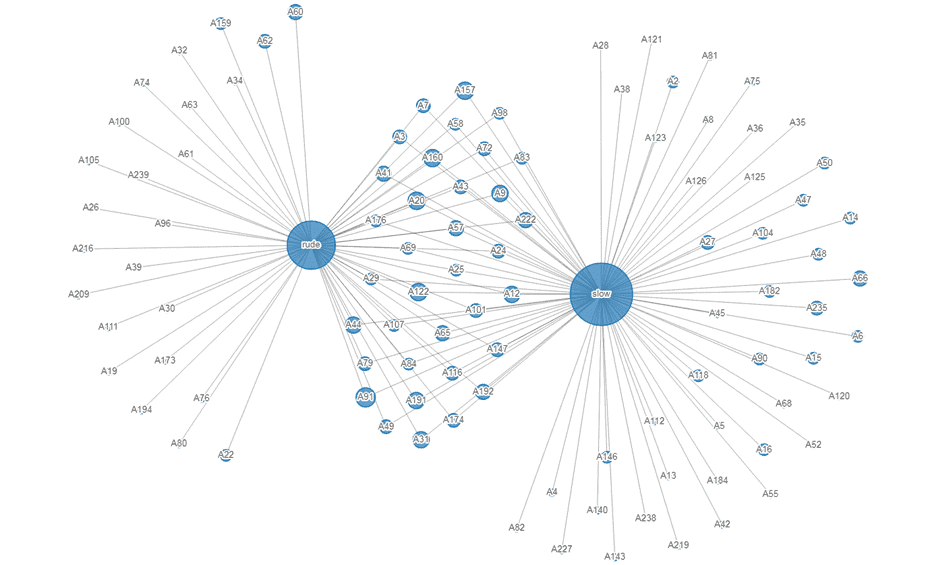
From this visualization, we can see the store numbers of the locations that have reviews that use the word “rude” or “slow”. The size of each circle (each circle without a word represents the stores) indicates how often those words appear. We can see there are a number of stores that struggle with both of these problems.
In summary, customer sentiment analysis can assist in creating relationships with customers and ensure that future consumers don’t have the same negative experience previous consumers might have had. It can be instrumental in quickly identifying not just an occurring problem, but where it persists to implement corrective actions quickly. This AI-enabled holistic customer experience approach can be a key step in recovering and increasing revenue, trustworthiness, and profitability.
Looking for more on Data & Analytics?
Explore more insights and expertise at smartbridge.com/data
There’s more to explore at Smartbridge.com!
Sign up to be notified when we publish articles, news, videos and more!
Other ways to
follow us: