The Strategic Value of Data-Centric Artificial Intelligence
Artificial Intelligence is a prevalent part of our lives, and as we progress forward, it’s essential that we optimize our approach to developing it. Rather than focus on the models, we should be focusing on feeding it good data. A data-centric AI method will lead to faster development, increased accuracy, and higher return on value.
You’re trying to make better tasting food by investing in new stoves, knives, sous vides, etc., pretty cool state-of-the-art stuff; Yet all along you’ve been cooking your dishes with low-quality ingredients, some of your ingredients are even expired!
Yeah, maybe those shiny new appliances are better than the old ones (marginally), but was the return-on-investment worth it? You could have spent much less and achieved much better results if you had focused on fresh, high-quality ingredients instead.
Oh, right, this is an artificial intelligence (AI) article. Sorry, just replace “kitchen tools” with AI / machine learning, and “ingredients” with data. The analogy holds true: Garbage data in –> Garbage results out.
What I’m trying to say is investing in improving quality data more often than not has a greater effect than investing in your data scientists and ML engineers tuning and retuning models for performance gains.
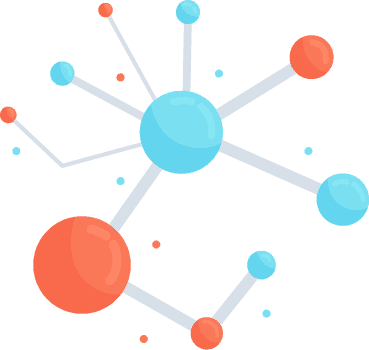
Focus on data quality all the way from source (data producers / recorders / application telemetry) through stream (data transformation and movement through data pipelines) and the AI you’ve already invested in will serve you better; Not to mention that your more classic analytics will become more trustworthy along the way.
Artificial intelligence (AI) is a topic of discussion we often hear in business innovation, but what does that mean for the data science measures that have already taken place in many organizations? More importantly with many of these technologies having specific costs associated with them, how do business decision-makers assess the value of decisions that relate to technology innovation such as AI and data science? We will discuss all this and more as we dive deeper into the value of data-centric artificial intelligence.
What is Data-Centric Artificial Intelligence?
Traditionally, data science and machine learning have focused on model architecture, algorithm design, and feature engineering. Data was often treated as a static artifact. Data-centric AI flips this approach, making data the primary focus for developing solutions that drive business results. It seeks better and faster ways to access and automate data processing workflows to generate valuable insights.
Data-centric AI can be used in predictive analytics to analyze and forecast trends in the data to make decisions. This type of AI leverages the data it gathers for various purposes, including generating predictions and making data-driven decisions.
Traditionally, data science and machine learning have primarily put emphasis on feature engineering, algorithm design, and bespoke model architecture for the purposes of modeling. Data is often treated as a static point-in-time artifact, and the bulk of the team’s focus is on the model itself. Streaming analytics has become a major part of the data architecture for organizations across industries and geographic locations. Model-centered AI often focuses on how a particular model can be changed to get different results. However, data-centered AI often makes data the mutable input or labels, creating vastly different outcomes while leaving the model consistent.
As the complexity of models continue to increase over time, it is more challenging than ever to find a model that is explainable, easy to deploy, and provides the accurate outcomes necessary for the hypothesis being posed within the business. Depicted in the image below, the conventional approach is to adjust the code and/or algorithms before attempting to change the data/variables being measured in the approach. However, this approach doesn’t leave enough space for inconsistent data that is typically present in large organizations where data cleansing is not governed or managed to provide a consistent framework to model from.
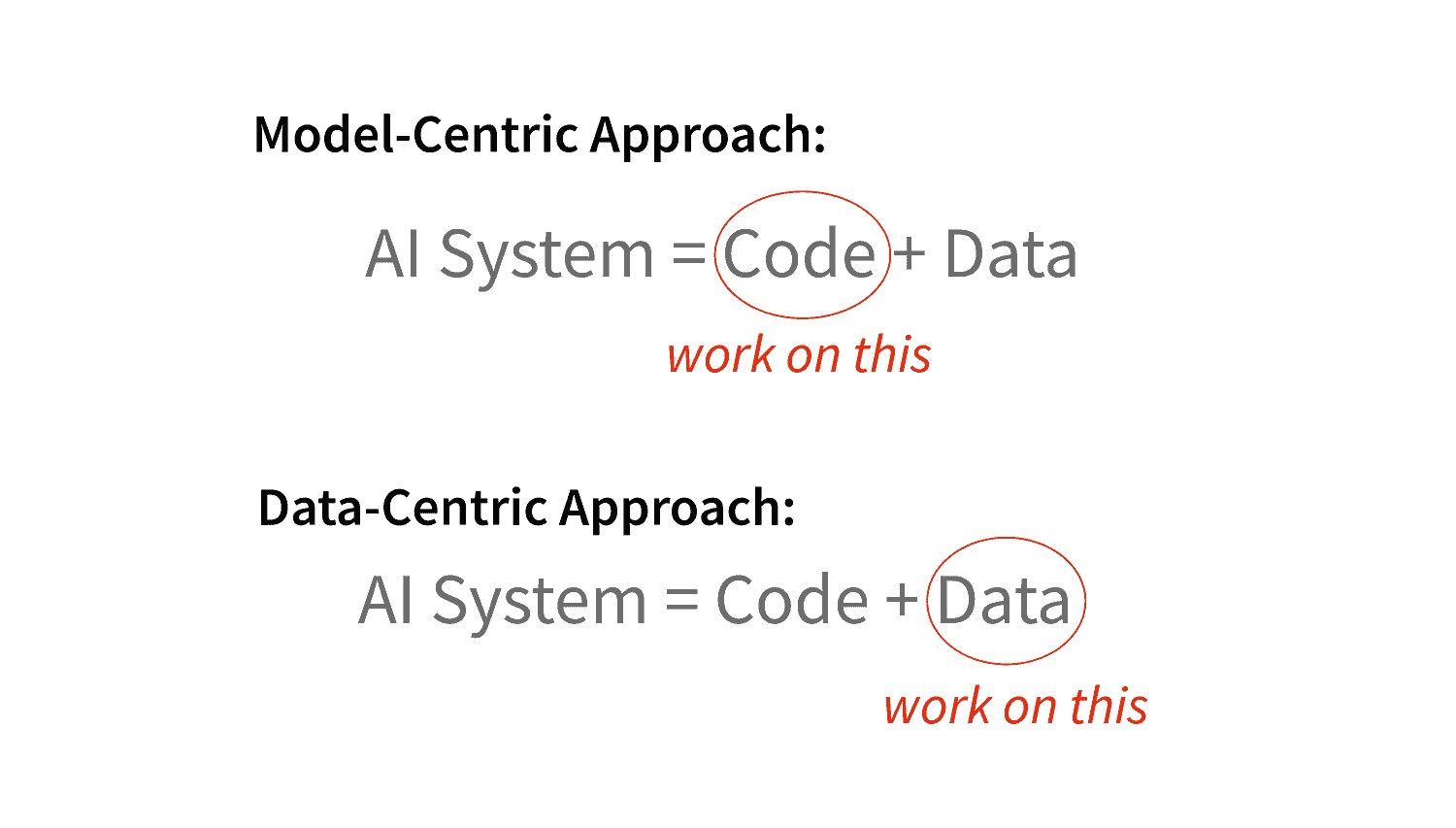
Data-centric AI vs Model/Code-centric AI Approach. Source
Why is Data-Centric AI Important?
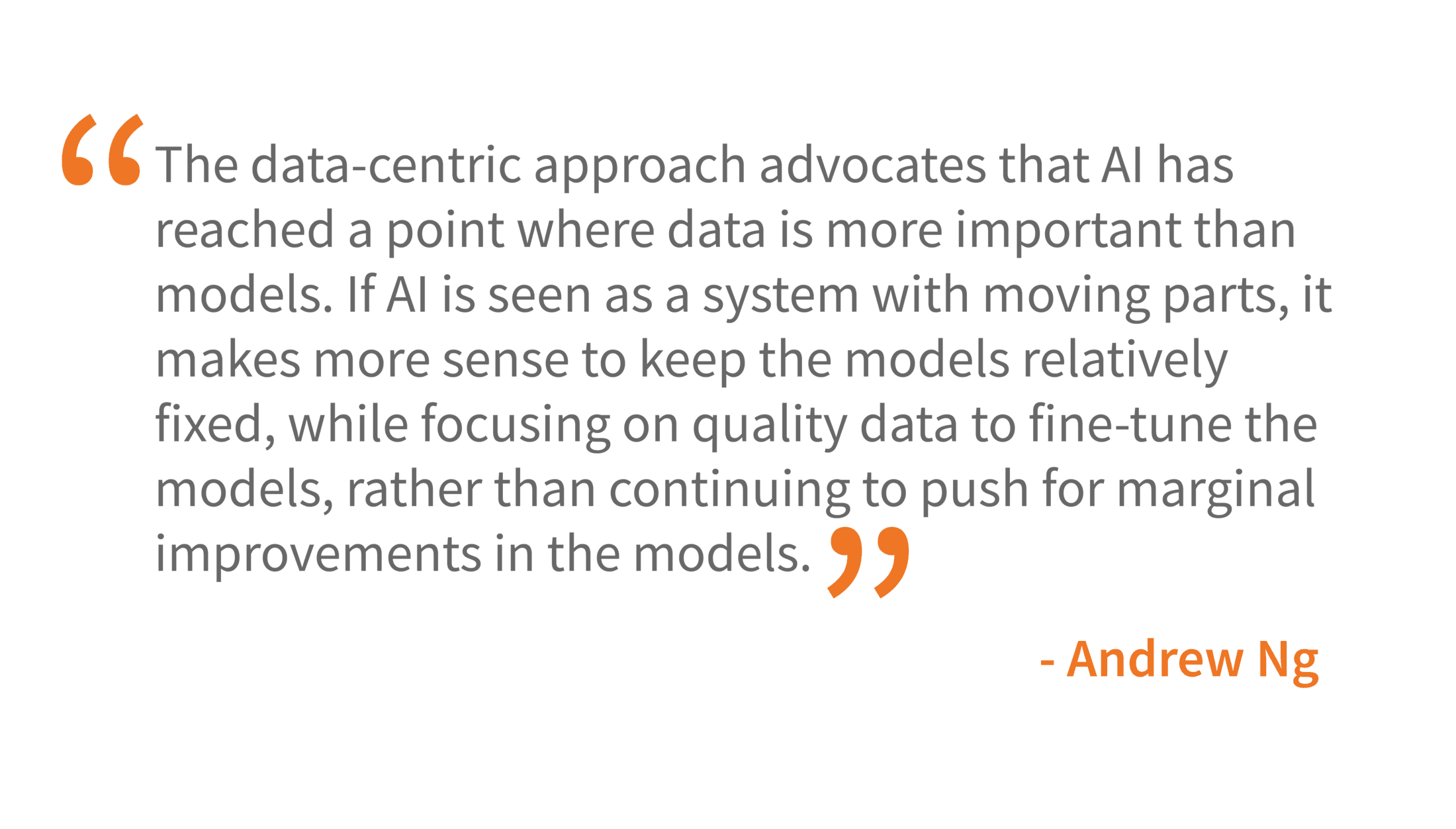
Which Businesses Benefit the Most From Data-Centric AI?
Data-centric AI can benefit any organization looking to better manage and leverage its data to make improved business decisions. Organizations with large data sets, such as financial services, healthcare, retail, and telecommunications, can benefit the most because they have more data to deal with and are looking for ways to optimize it.
The use cases are endless. It can be used for anything from predicting customer behavior to predicting the stock market. It is not limited by the constraints of human intelligence and can process much more information than a human being could. It can also learn from its mistakes and improve itself over time.
Challenges of Implementing Data-Centric AI
How Do We Measure the Value of Data-Centric AI?
Organizations can use a variety of methods to determine the value of data-centric AI. One of the most common ways to measure the value is by calculating the cost savings related to the optimization of existing processes. For example, if an organization implements data-centric AI solutions to streamline the process of generating reports for customers, then the organization can measure the value by calculating the cost savings of not manually creating the reports.
Alternatively, businesses could measure the impact of data-centric AI solutions from their top and/or bottom line, assigning value to the outcomes achieved alongside any larger qualitative goals. Data and analytics can measure the results of project initiatives, but the success of these projects often relies on the presence of champions within the organization. These internal champions keep their users engaged and help business leaders understand the overall impact of changes made within the organization. The steps below provide a simple way for organizations to measure the ROI or Return on Value (ROV) of data-centric AI. These steps are also applicable to a variety of organizational-level, technology-focused, digital transformation efforts.
Step 1: Frame the business problem and define measurable outcomes for success
Projects need clearly identified business problems in order to determine what the success of a project looks like. From there, it is important to know how to best measure success by utilizing the SMART goal framework, which stresses goals should be both measurable and time-specific. Organizational leadership should also decide if the goals are easily measurable based on the data currently available. Measurements are often established with little consideration of how outcomes themselves will be measured based on the data currently available.
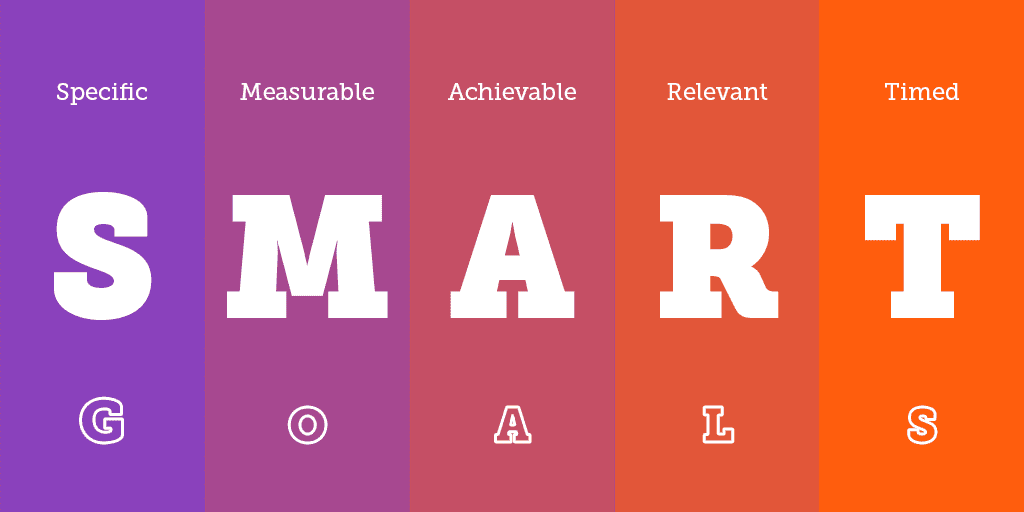
Step 2: Measure outcomes indicated by connecting data and analytics
Not only is data critical to AI, data is important to the overall success of the entire organization, establishing analytics to measure the outcomes indicated in the previous step. This is critical to the success of the current business problem as well as any subsequent problems encountered. In order to scale in a way that’s measurable and intentional, businesses should ensure they take a data-centric approach to measuring value-driven outcomes.
Step 3: Determine a breakeven point (B/E) based on TCO as well as qualitative and quantitative values
Total Cost of Ownership (TCO) is the cost of any technology adoption and/or associated subscriptions. This value is often tracked for budgeting purposes and reported as part of critical decision-making. However, this TCO number should be presented alongside measurable outcomes to determine a breakeven point (B/E) for the organization’s financial outlook. Most companies focus on qualitative value from technology innovation, and they do not take the time to establish quantitative measures that business leaders can easily attribute revenue enablement activities to the budget allotted to TCO.
Following the steps above ensures that data-centric AI planning and decision-making is focused on the overall value associated with the specific outcomes identified by business stakeholders that are critical to organizational success. Additionally, we recommend starting with a proof of concept (POC) project to get initial investment from senior leadership towards a larger more concentrated data-centric AI effort. These projects can take anywhere from five to eight weeks depending on the size of the organization and scope of the project. A POC can enable your organization to quickly test assumptions and drive specific measurable outcomes that often make it easier to engage in further discussions around what is possible.
The Future of Data-Centric AI
Data-centric AI is the future of AI development. By prioritizing data quality and taking a data-driven approach, organizations can unlock the full potential of AI and achieve significant business benefits.
Quickly Scale Data-Centric AI with Power BI
Power BI is a powerful tool with hundreds of interactive visualizations at your disposal to build reports and dashboards showcasing AI-driven business analytics and insights. Coupled with Azure Synapse Analytics,, Power BI can connect across all of your data sources, wherever they sit, no matter the size or complexity of the systems. Within the new Microsoft Teams Power BI app, you can quickly discover and combine datasets across systems and departments to provide actionable insights, collaborate with others, and make more personal, informed decisions.
Updates include:
Power BI continues to bridge the gap between data and decisions to drive innovation.
Keep Reading: Artificial Intelligence vs. Intelligent Assistance
Looking for more on AI/ML?
Explore more insights and expertise at smartbridge.com/ai
There’s more to explore at Smartbridge.com!
Sign up to be notified when we publish articles, news, videos and more!
Other ways to
follow us: