AI/ML Case Study
Device Tracking Improvements with Azure Machine Learning Implementation for a Global Med Device Company
Client
The client is a global medical technology company specializing in the development of innovative solutions for treating cardiovascular diseases and neuromodulation disorders. Their product portfolio focuses on improving patient outcomes in areas such as cardiac surgery, neuromodulation for epilepsy, and sleep apnea therapy. With operations across more than 100 countries, the company aims to advance healthcare through cutting-edge technology and research.
Project Scope
Current tracking processes are not as thorough
Since the company is a global company, they need to ship devices all over the world. Each device needs to be tracked for various reasons including compliance, however, with their current system, they are not able to completely and thoroughly track the devices as well as they could especially when there are anomalies. The shipping data comes from disparate sources, from different systems and at different points in time. The client would like to bring them all together to create one combined shipping database that can then be used in reports, dashboards, etc.
The client needed to migrate their data and processes to the cloud, so they can scale their operations, securely access the data they needed when they needed it and improve existing pipelines and establish new ones. Therefore, the main objective for them is to move their data science workloads to the Azure Machine Learning (Azure ML) platform. Azure ML addresses traditional machine learning challenges by offering automated processes, robust data security, and scalable cloud infrastructure.
Key Challenges
A local system and manual handling of data creates hurdles
The Smartbridge Solution
Implementing an enterprise-level Azure Machine Learning program
The Smartbridge team used MLOps (Machine Learning Operations) practices to help the client enable collaboration and streamline their processes. MLOps is a set of practices that combine machine learning, DevOps, and data engineering principles to automate and standardize the development, deployment, and monitoring of machine learning models. By implementing MLOps, the team ensured that the machine learning lifecycle—covering model development, testing, deployment, and ongoing monitoring—was fully integrated into a scalable and efficient process.
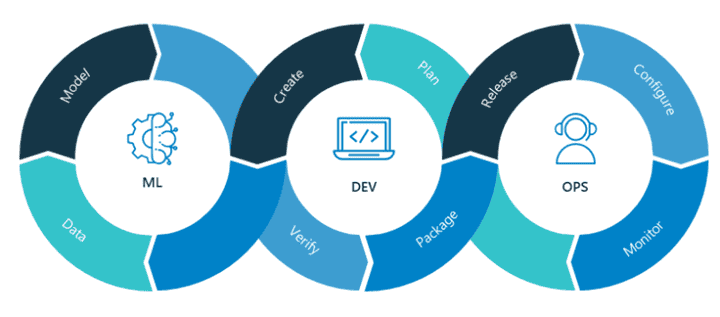
The team started by transitioning disparate data science workloads to the Azure machine learning platform, which not only enabled seamless integration, scalability, and security but also provided access to a wide range of tools and services necessary for efficient model management.
In addition to migrating to Azure ML, the team re-factored the project to align with the ML lifecycle, making the solution monitorable, maintainable, and adaptable to future changes. By building a pipeline and organizing each step as an individual block, the team ensured that the system is highly modular. This modularity offers several benefits:
When using Azure, Microsoft recommends using Python, but as previously stated, the client’s data science team uses R as their programming language. Smartbridge solved this discrepancy by creating a Python wrapper to go around the R code, so the client can still use codes they previously created and feel comfortable continuing using the language they are used to until they can pick up Python.
To resolve the issue of security, the team put the data in a secured storage container and create a secured connection to the Azure ML environment. This eliminates the need for data to be downloaded to local machines in order to be used allowing the company to stay in full compliance with corporate and healthcare policies.
Finally, Smartbridge was also able to implement versioning for models which allowed the client to retrain models without losing their original versions, so they can do things like compare and validate results.
Overall Solution:
Prior to the Smartbridge solution, the data science team was restricted to sending the data to its stakeholders and letting them figure out the anomalies. In addition, identifying an anomaly in the shipping process and finding who to talk to about it was a manual, repetitive process. Now with all of the data aggregated in one place with valuable insights, business users are able to access the information they need without having to waste time tracking it down.
Value Delivered
Creating a 87% reduction in processing time
Without Azure machine learning, the anomaly detection process could take nearly an entire workday. With an automation pipeline in Azure ML, it would take less than an hour.
There’s more to explore at Smartbridge.com!
Sign up to be notified when we publish articles, news, videos and more!
Other ways to
follow us: