The Difference Between Data Science vs Data Analytics
Data science and data analytics are both terms that have become heavily used these days, but what’s the difference between them? We’ll examine what each of these terms mean, and how they can both be applied to business processes together to aid in digital innovation.
Data-driven intelligence is embedded in our lives. Businesses are constantly changing how they interact with data to provide value for their customers in addition to changing their internal processes to be more efficient and agile. Data analytics and data science can help extract business value from data. We’ll dive into the similarities, differences, and evolution of data science and data analytics.
Data Analytics
Data analytics is defined as the process of examining raw data to identify trends and draw conclusions about that information. Most data analytics techniques have been automated into processes and algorithms using software systems that work over raw data to generate valuable information.
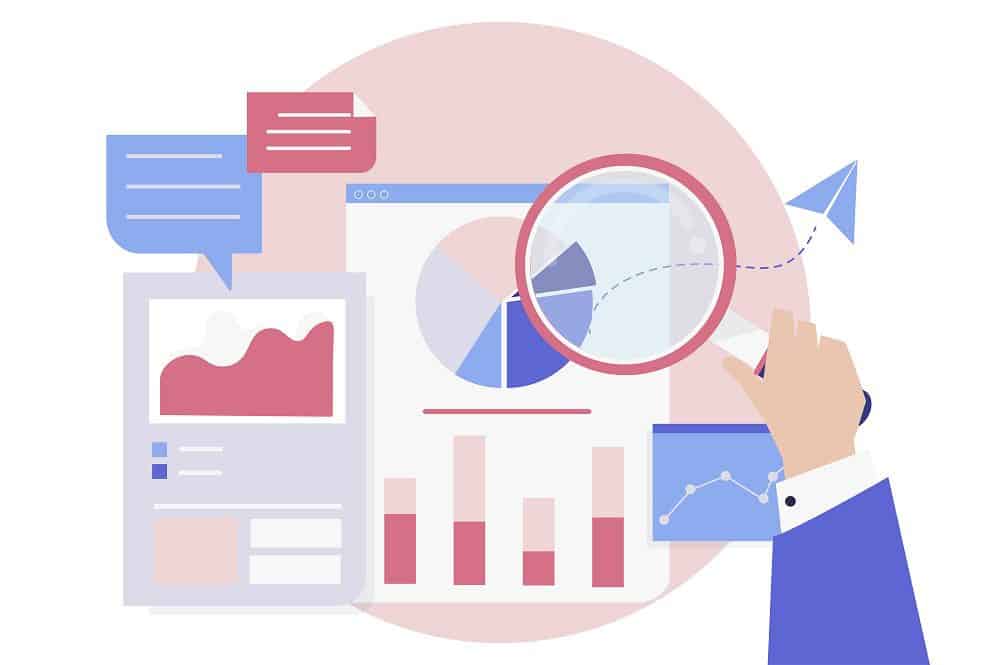
For the last few decades, data analytics has been used by many industries such as financial services, retail, insurance, airline, energy trading, etc. The list goes on and on, but some widespread examples include credit risk assessment used by banks, risk-based insurance premium determination, optimal ticket price for airlines, and electricity pricing in unregulated markets.
These use-cases are driven by algorithms that were written to balance supply-demand or meet certain business criteria to maximize business profitability. However, these analytics mostly rely on small amounts of highly relevant data, typically using on-premise data storage and computation. Some of the business processes that rely on these algorithms can be manually intensive with many human touchpoints. The algorithm development part of the aforementioned process relies on data science.
Data Science
Data science constitutes a multi-disciplinary approach of combining different fields-mathematics, statistics, and programming aided by computation to interpret massive amounts of data for decision-making purposes.1 Data science uses techniques, machine learning (ML) and artificial intelligence (AI), to extract meaningful information and predict future patterns and behaviors.
Referring to the examples mentioned above, data science deals with methods such as logistic regression to determine the default risk for a financial service provider. Similarly, airlines use data science methods such as the random forest algorithm (supervised algorithm) to provide optimal pricing.
In summary, data science methods and data analytics have been used for the last four decades to drive business outcomes across various industries. However, their prominence and popularity have increased significantly in the last decade.
Newer Trends
1. The rise of smartphones, wearables, IoT (Internet of things), advancements in technology, internet, and social media have resulted in a lot more data being created and has allowed easier access to big data.
2. Cloud adoption (managed storage services) has gained a lot of market share, and the marginal cost of storage has fallen by 75 percent in the last 5 years.¹
3. The ability to use the abundance of data we are constantly gathering to develop deeper insights using pay-as-you-go software service models has helped businesses realize value from their data quickly.
4. Market forces such as Google, Amazon, and Uber have showcased data-driven business transformation and disrupted a lot of traditional industries/processes by putting the customer at the center of their business.
All of these factors result in the acceleration and massive scale-up of data analytics and data science-driven transformation. This also has led to the development and wide-spread use of sophisticated AI/ML techniques such as reinforcement learning, deep learning, neural networks, and others.
Current State Data-Driven Use Cases
Data science and data analytics are creating intelligent and efficient businesses, which are led by human-assisted AI in advanced cases. Data-driven companies leverage a data platform-centered approach that encompasses data ingestion, data labeling, data governance, data engineering, algorithm development, algorithm refinement, insights-driven business process development, and business value realization. Based on that understanding, let’s revisit the use cases discussed above in the current state.
Conclusion
In conclusion, data-driven business execution (using data analytics and data science) is not new; however, the amount of data used to arrive at the conclusions and the pace of change has increased drastically. Smartbridge has 19 years of experience assisting our clients in their data journey. If you would like to learn how data science and data analytics can help propel your business, contact us today.
References
- Storage Technology Costs Declining But Business Demand Drives Total Spending (datastorageconnection.com)
- Microsoft and Meta join Google in using AI to help run their data centers | TechCrunch
- Microsoft Customer Story-Walgreens empowers pharmacists with an intelligent prescription data platform on Azure
Looking for more on Data Analytics or Data Science?
Explore more insights and expertise at smartbridge.com/data and smartbridge.com/ai
There’s more to explore at Smartbridge.com!
Sign up to be notified when we publish articles, news, videos and more!
Other ways to
follow us: